From the islands of Ibiza to Germany's Wetterstein Mountains, these amazing hotel suites set the bar for modern luxury travel.
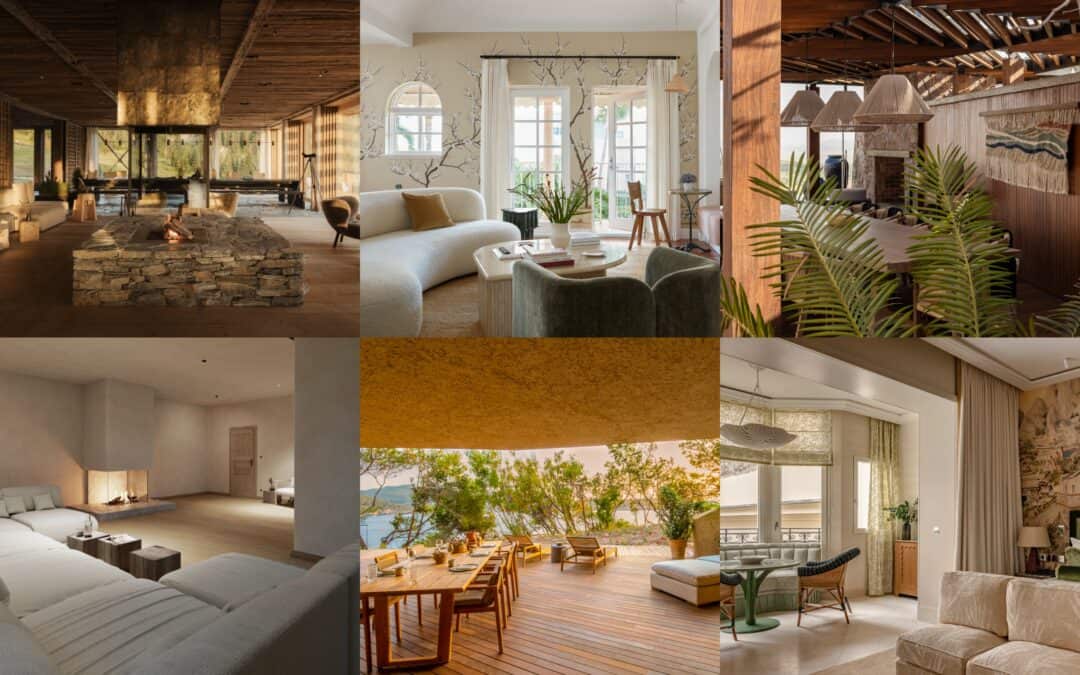
From the islands of Ibiza to Germany's Wetterstein Mountains, these amazing hotel suites set the bar for modern luxury travel.
All aboard a seven-night stay on Virgin Voyages' Valiant Lady, boasting luxury accommodations, excellent amenities and more.